Moderating Harassment in Twitter with Blockbots
By Stuart Geiger, ethnographer and post-doctoral scholar at the Berkeley Institute for Data Science | Permalink
I’ve been working on a research project about counter-harassment projects in Twitter, where I’ve been focusing on blockbots (or bot-based collective blocklists) in Twitter. Blockbots are a different way of responding to online harassment, representing a more decentralized alternative to the standard practice of moderation — typically, a site’s staff has to go through their own process to definitively decide what accounts should be suspended from the entire site. I’m excited to announce that my first paper on this topic will soon be published in Information, Communication, and Society (the PDF on my website and the publisher’s version).
This post is a summary of that article and some thoughts about future work in this area. The paper is based on my empirical research on this topic, but it takes a more theoretical and conceptual approach given how novel these projects are. I give an overview of what blockbots are, the context in which they have emerged, and the issues that they raise about how social networking sites are to be governed and moderated with computational tools. I think there is room for much future research on this topic, and I hope to see more work on this topic from a variety of disciplines and methods.
What are blockbots?
Blockbots are automated software agents developed and used by independent, volunteer users of Twitter, who have developed their own social-computational tools to help moderate their own experiences on Twitter.
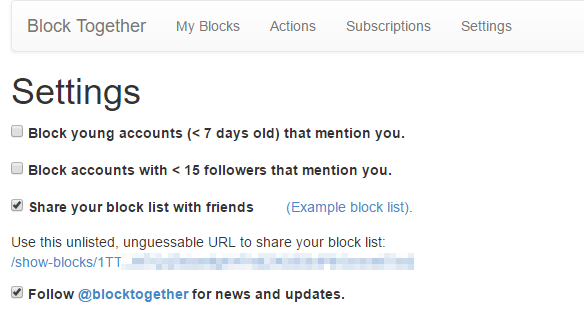
The blocktogether.org interface, which lets people subscribe to other people’s blocklists, publish their own blocklists, and automatically block certain kinds of accounts.
Functionally, blockbots work similarly to ad blockers: people can curate lists of accounts they do not wish to encounter, and others can subscribe to these lists. To subscribe, you have to give the blockbot limited access to your account, so that it can update your blocks based on the blocklists you subscribe to. One of the most popular platforms for supporting blockbots in Twitter is blocktogether.org, which is what hosts the popular ggautoblocker project and many more smaller, private blocklists. Blockbots were developed to help combat harassment in the site, particularly coordinated harassment campaigns, although they are a general purpose approach that can be used to filter across any group or dimension. (More on this later in this post.)
A subscription-based model
Blockbots extend the functionality of the social networking site to make the work of responding to harassment more efficient and more communal. Blockbots are based on the standard feature of individual blocking, in which users can hide specific accounts from their experience of the site. Blocking has long been directly integrated into Twitter’s user interfaces, which is necessary because by default, any user on Twitter can send tweets and notifications to any other user. Users can make their accounts private, but this limits their ability to interact with a broad public — one of the big draws of Twitter versus more tightly bound social networking sites like Facebook.
For those who wish to use Twitter to interact with a broad public and find themselves facing rising harassment, abuse, trolling, and general incivility, the typical solution is to individually block accounts. Users can also report harassing accounts to Twitter for suspension, but this process has long been accused of being slow and opaque. People also have quite different ideas about what constitutes harassment, and the threshold to get suspended from Twitter is relatively high. As a result, those who are facing unsolicited remarks find themselves facing a Sisyphean task of individually blocking all accounts that send them inappropriate mentions. This is a large reason why a subscription model has emerged in bot-based collective blocklists.
Some blockbots use lists curated by a single person, others use community-curated blocklists, and a final set use algorithmically-generated blocklists. The benefit of using blockbots is that it lets ad-hoc groups form around common understandings of what they want their experiences to be on the site. Blockbots are opt-in, and they only apply for the people who subscribe to them. There are groups who coordinate campaigns against specific individuals (I need not name specific movements, but you can observe this by reading the abusive tweets received in just one week by Anita Sarkeesian of Feminist Frequency, many of which are incredibly emotionally disturbing). With blockbots, the work of responding to harassers can be efficiently distributed to a group of likeminded individuals or delegated to an algorithmic process.
Blockbots first emerged in Twitter in 2012, and their development followed a common trend in technological automation. People who were a part of the same online community found that they were being harassed by a common set of accounts. They first started sharing these accounts manually whenever they encountered them, but they found that this process of sharing blockworthy accounts could be automated and made more collective and efficient. Since then, the computational infrastructure has grown in leaps and bounds. It has been standardized with the development of the blocktogether.org service, which makes it easy and efficient for blocklist curators and subscribers to connect. People do not need to develop their own bots anymore, they only need to develop their own process for generating a list of accounts.
How are public platforms governed with data and algorithms?
Beyond the specific issue of online harassment, blockbots are an interesting development in how public platforms are governed with data and algorithmic systems. Typically, the responsibility for moderating behavior on social networking sites and discussion forums falls to the organizations that own and operate these sites. They have to both make the rules and enforce them, which is increasingly difficult to do at the scale that many of these platforms now have achieved. At this scale, not only is there a substantial amount of labor involved to do this moderation work, but it is also increasingly unlikely to find a common understanding about what is acceptable and unacceptable. As a result, there has been a proliferation of flagging and reporting features that are designed to collect information from users about what material they find inappropriate, offensive, or harassing. These user reports are then fed into a complex system of humans and algorithmic agents, who evaluate the reports and sometimes take an action in response.
I can’t write too much more about the flagging/reporting process on the platform operator’s side in Twitter, because it largely takes place behind closed doors. I haven’t found too many people who are satisfied with how moderation takes place on Twitter. There are people who claim that Twitter is not doing nearly enough to suspend accounts that are sending harassing tweets to others, and there are people who claim that Twitter has gone too far when they do suspend accounts for harassment. This is a problem faced by any centralized system of authority that has to make binary decisions on behalf of a large group of people; the typical solution is what we usually call “politics.” People petition and organize around particular ideas about how this centralized system ought to operate, seeking to influence the rules, procedures, and people who make up the system.
Blockbots are a quite different mode of using data and algorithms to moderate large-scale platforms at scale. They are still political, but they operate according to a different model of politics than the top-down approach that places a substantial responsibility for governing a site on platform operators. In my studies of blockbot projects, I’ve found that members of these groups have serious discussions and debates about what kind of activity they are trying to identify and block. I’ve even seen groups fracture and fork over different standards of blockworthyness — which I think can sometimes be productive. A major benefit of blockbots is that they do not operate according to a centralized system of authority where there is only one standard of blockworthyness, such that someone is either allowed to contact anyone or no one.
Blockbots as counterpublic technologies
In the paper, I analyze blockbot projects as counterpublics, taking from the term Nancy Fraser coined in her excellent critique of Jurgen Habermas’s account of the public sphere. Fraser argues that there are many publics where people assemble to discuss issues relevant to them, but only a few of these publics get elevated to the status of “the public.” She argues that we need to pay attention to the “counterpublics” that are created when non-dominant groups find themselves excluded from more mainstream public spheres. Typically, counterpublics have been analyzed as “separate discursive spaces:” safe spaces where members of these groups can assemble without facing the chilling effects that are common in public spheres. However, blockbots are a different way of parallelizing the public sphere than ones that have been historically analyzed by scholars of the public sphere.
One aspect of counterpublics is that they serve as sites of collective sensemaking: they are a space where members of non-dominant groups can work out their own understandings about issues that they face. I found a substantial amount of collective sensemaking in these groups, which can be seen in the intense debates that sometimes take place over defining standards of blockworthyness. As a blockbot can be easily forked (particularly with the blocktogether.org service), people are free to imagine and implement all kinds of possibilities about how to define harassment or any other criterion. People can also introduce new processes for curating a blocklist, such as adding a human appeals board for a blocklist that was generated by an algorithmic process. I’ve also seen a human curated blocklist move from a “two eyes” to “four eyes” principle, requiring that every addition to a blocklist be approved by another authorized curator before it would be synchronized with all the subscribers.
Going beyond “What is really harassment?”
Blockbots were originally created as counter-harassment technologies, but harassment is a very complicated term — one that even has different kinds of legal significance in different jurisdictions. One of the things I have found in conducting this research is that if you ask a dozen people to define harassment, you’ll get two dozen different answers. Some people who have found themselves on blocklists have claimed that they do not deserve to be there. And like in any debate on the Internet, there have even been legal threats made, including those alleging infringements of freedom of speech. I do think that the handful of major social networking sites are close to having a monopoly on mediated social interaction, and so the decisions they make about who to suspend or ban are ones we should look at incredibly closely. However, I think it is important to acknowledge these multiple definitions of harassment and other related terms, rather than try and close them down and find one that will work for everyone.
I think it is important and useful to move away from having just one single authoritative system that returns a binary decision about whether an activity is or is not allowed for all users of a site. I’ve seen controversies over this not just with harassment/abuse/trolling on Twitter, but also with things like photos of breastfeeding on Facebook. I think we should be exploring tools to give people more agency over moderating their own experiences on social networking sites, where ‘better’ means both more efficiently and more collectively. Facebook already uses sophisticated machine learning models to try and intuit what it thinks you want to see (i.e. what will keep you on the site looking for ads the longest), but I’d rather see this take place in a more deliberate and transparent manner, where people take an active role in defining their own expectations.
I also think it is important distinguish between the right to speak and the right to be heard, particularly in privately-owned social networking sites. Being placed on a blocklist means that someone’s potential audience is cut, which can be a troubling prospect for people who are used to their speech being heard by default. In the paper, I do discuss how modes of filtering and promotion are the mechanisms in which cultural hegemony operates. Scholars who focus on marginalized and non-dominant groups have long noted the need to investigate such mechanisms. However, I also review the literature about how harassment, trolling, incivility, and related phenomena are also ways in which people are pushed out of public participation. The public sphere has never been neutral, although the fiction that it is a neutral space where all are on equal group is one that has long been advanced by people who have a strong voice in such spaces.
How do you best build a decentralized classification system?
One issue that is relevant in these projects is about the kind of false positive versus false negative rates we comfortable having. No classification system is perfect (Bowker and Star’s Sorting Things Out is a great book on this), and it isn’t hard to see why someone facing a barrage of unwanted messages might be more willing to face a false positive than a false negative. On this issue, I see an interesting parallel with Wikipedia’s quality control systems, which my collaborators and I have written extensively about. There was a point in time when Wikipedians were facing a substantial amount of vandalism and hate speech in the “anyone can edit” encyclopedia, far too much for them to tackle on their own. They developed a lot of sophisticated tools (see The Banning of a Vandal and Wikipedia’s Immune System). However, my collaborators and I found that there are a lot of false positives, and this can inhibit participation among the good-faith newcomers who get hit as collateral damage. And so there have been some really interesting projects to try and correct that, using new kinds of feedback mechanisms, user interfaces, and Application Programming Interfaces (like Snuggle and ORES, led by Aaron Halfaker).
I suspect that if this decentralized approach to moderation in social networking sites gets more popular, then we might see a whole sub-field emerge around this issue, extending work done in spam filtering and recommender systems. Blockbots are still at the initial stages of their development, and think there is a lot of work still to be done. How do we best design and operate a social and technological system so that people with different ideas about what constitutes harassment can thoughtfully and reflectively work out these ideas? How do we give people the support that they need, so that responding to harassment isn’t something people have to do on their own? And how can we do this at scale, leveraging computational techniques without losing the nuance and context that is crucial for this kind of work? Thankfully, there are lots of dedicated, energetic, and bright people who are working on these kinds of issues and thinking about these questions.
Personal issues around researching online harassment
I want to conclude by sharing some anxieties that I face in publishing this work. In my studies of these counter-harassment projects, I’ve seen the people who have taken a lead on these projects become targets themselves. Often this stays at the level of trolling and incivility, but it has extended to more traditional definitions of harassment, such as people who contact someone’s employer and try to get them fired, or people who send photos of themselves outside of that person’s place of work. In some cases, it becomes something closer to domestic terrorism, with documented cases of people who have had the police come to their house because someone reported a hostage situation at their address, as well as people who have had to cancel presentations because someone threatened to bring a gun and open fire on their talk.
Given these situations, I’d be lying if I said I wasn’t concerned that this kind of activity might come my way. However, this is part of what the current landscape around online harassment is like. It shows how significant this problem is and how important it is that people work on this issue using many methods and strategies. In the paper, I spend some time arguing why I don’t think that blockbots are part of the dominant trend of “technological solutionism,” where a new technology is celebrated as the definitive way to fix what is ultimately a social problem. The people who work on these projects don’t talk about them in this solutionist way either. However, blockbots are tackling the symptoms of a larger issue, which is why I am glad that people are working on multifaceted projects and initiatives that investigate and tackle the root causes of harassment, like HeartMob, Hollaback, Women, Action, and the Media, Crash Override, the Online Abuse Prevention Initiative,, the many researchers working on harassment (see this resource guide), the members of Twitter’s recently announced Trust and Safety Council, and many more people and groups I’m inevitably leaving out.
Cross-posted to the Berkeley Institute for Data Science.
No Comments